Statistical Risk Calibration: Shielding Momentum Portfolios from Crash Risk
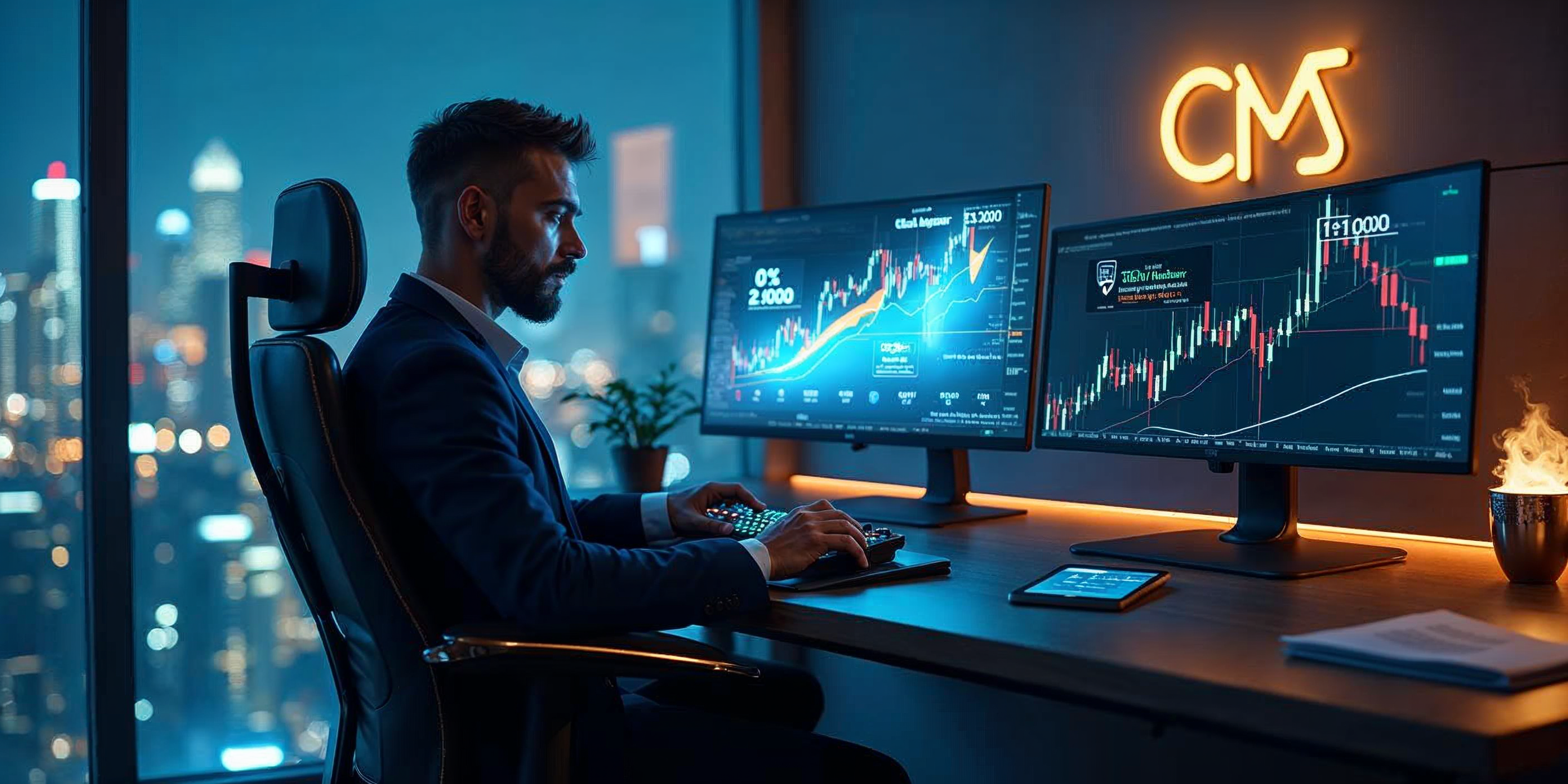
Overview
Momentum is famous for its returns—and infamous for its drawdowns. 2009, 2018, 2020 and late-2023 all witnessed abrupt reversals that erased months of gains in days. The antidote is not to abandon momentum but to engineer a risk engine that senses turbulence, scales exposure, and steers the portfolio away from regime cliffs.
1. Measuring Volatility the Right Way
Daily standard deviation is a blunt proxy; it misses intraday jumps and volatility clustering. Modern desks deploy a volatility stack:
- Realised Variance from five-minute bars; robust to microstructure noise.
- EWMA Volatility with decay λ chosen via minimum forecast-error.
- GARCH(1,1) to anticipate tomorrow’s σ²; crucial for overnight margin limits.
A 2024 SSRN study shows that simple volatility scaling — setting weight = target risk / estimated σ — lifts Sharpe by 50 % in crypto and in equity momentum baskets alike.
2. Drawdown & Tail-Risk Metrics
Volatility captures dispersion, but momentum dies from skew.
Key metrics:
Metric | What it Captures | Edge for Momentum |
Conditional VaR (CVaR) | Expected loss beyond α-quantile | Directly guards against crash tails |
Conditional Drawdown (CDD) | Mean of worst drawdowns over horizon | Better aligns with investor pain |
Max Drawdown Speed | Ratio of depth to time | Flags fast reversals typical of crowded trades |
Research on maximum drawdown–based ranking rules finds they out-perform classic cumulative-return momentum precisely because they sidestep high-skew names.
3. Dynamic Position Sizing: Volatility Scaling & Regime Switching
Risk models convert statistics into actionable sizing. Two mechanisms dominate:
- Volatility Targeting: Position = k / σ̂. When 30-day σ spikes from 12 % to 24 %, exposure halves automatically.
- Momentum-Reversal Switch: If realised volatility crosses its 90th percentile, flip to a mean-reversion strategy that profits from unwinding crowding. A 2024 switching framework shows this beats plain scaled momentum by maintaining constant leverage while avoiding ex-post information.
What if volatility remains low but correlation spikes? Then volatility targeting alone fails — see next section.
4. Correlation-Aware Portfolio Construction
Momentum universes tend to cluster into tech, cyclicals, or small-caps, breeding correlated risk. A three-layer correlation defence works:
- Rolling Correlation Matrix (90-day): if average pair-wise ρ > 0.6, impose weight caps.
- PCA Factor Lens: allocate risk equally across first K principal components so no single factor exceeds 25 % tracking error.
- Dynamic Basket Hedging: short a sector ETF whose beta to the portfolio > 0.8; size to bring net beta < 0.3.
5. End-to-End Risk Engine Blueprint
- Data Ingestion – minute bars, corporate actions, option-implied vol surfaces.
- Statistical Core
- Volatility stack (realised, EWMA, GARCH).
- Tail stack (CVaR, CDD).
- Correlation engine (dynamic PCA).
- Decision Layer
- Exposure = min(vol-target weight, CVaR-target weight).
- If regime = high-vol, switch to reversal template; else remain in momentum.
- Execution & Monitoring
- Route via smart-order router that minimises implementation shortfall.
- Real-time PnL shock tester: simulate ±2σ price jump; verify portfolio loss < allowed max.
- Post-Trade Analytics
- Attribute slippage to spread, market impact, delay.
- Weekly “crash drill” replaying historical 2018 Q1 vol spike to ensure guardrails still hold.
6. Conclusion
Momentum’s Achilles heel is not false signals but unmanaged risk convexity. By embedding a statistical risk engine — one that fuses volatility scaling, tail-risk guards, and correlation control — traders convert momentum from a fair-weather friend into an all-terrain ally. The guiding “What if?” remains crucial: What if vol doubles overnight? What if the whole basket loads onto one factor? With the answers coded into dynamic position sizing and real-time limit checks, the strategy can harvest trend alpha while dodging the dreaded crash signature that has humbled so many momentum funds before.
Both articles together equip a momentum desk with a full-stack statistical toolkit: first, extract and validate genuine trends; second, calibrate and clip risk before it turns terminal. Combined, they offer a robust, adaptive pathway to sustainable momentum alpha.