Designing Factor Models for Trading Gold
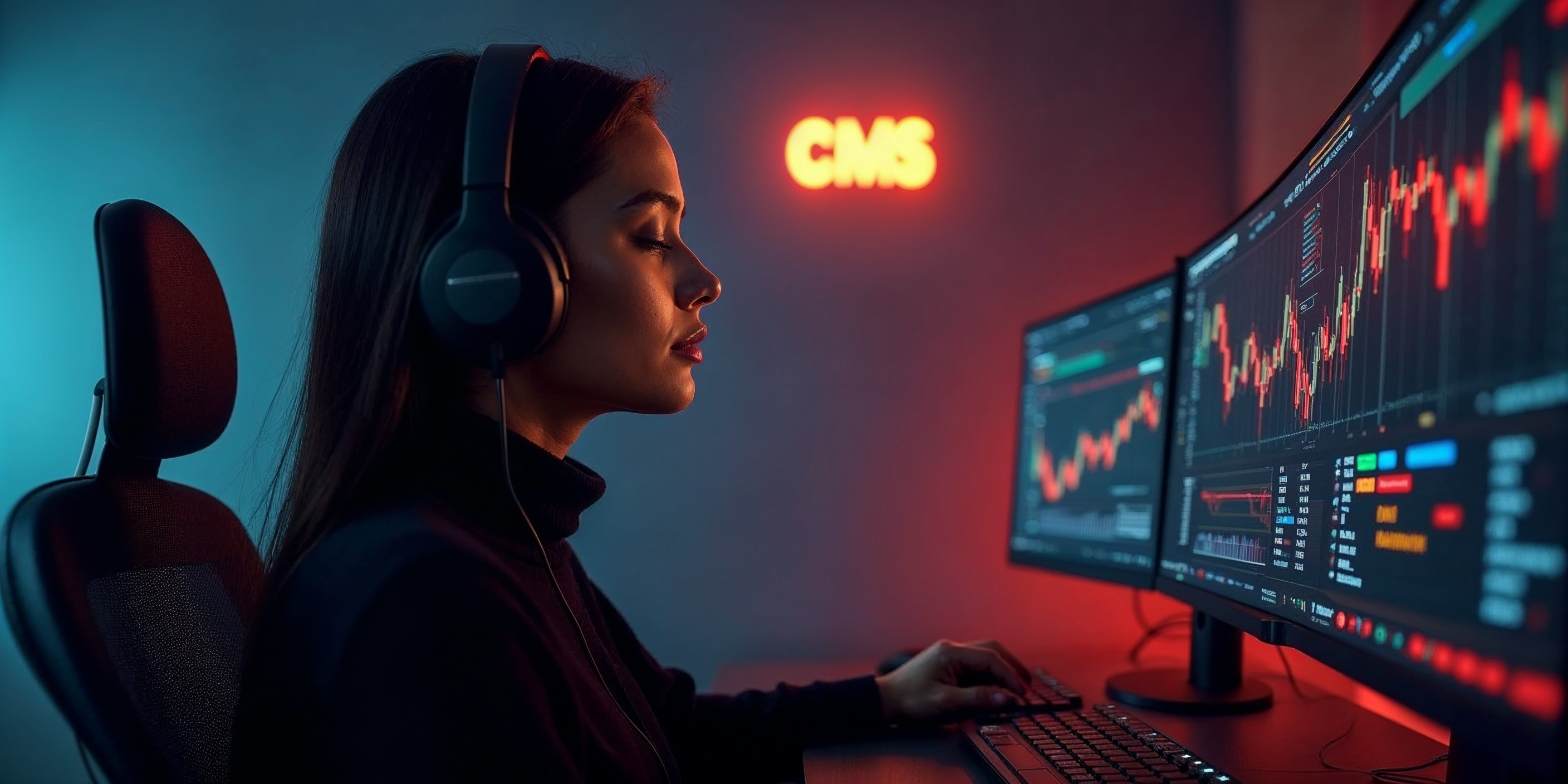
1. Introduction: Gold’s Multi-Dimensional Drivers
Gold is part currency, part commodity, part financial asset. Building a factor model therefore demands a tri-modal approach capturing:
- Macro-monetary variables (real yields, USD index, inflation expectations).
- Commodity-specific forces (mine supply disruptions, ETF flows, futures curve structure).
- Behavioral & hedging demand (central-bank purchases, retail fear gauges).
Gold’s liquidity and diverse usage make it ideal for systematic models—yet its idiosyncrasies (e.g., absence of a dividend or carry in the physical market) require bespoke factors.
2. Defining Gold Factor Categories
Factor | Concept | Construction Notes |
Real Interest-Rate Factor | Opportunity cost of holding non-yielding gold | −1 × 10-year TIPS yield, or OIS–breakeven inflation spread |
Dollar Strength Factor | FX translation effect | Inverse DXY, or weighted basket of top gold-consuming currencies (CNY, INR) |
Inflation & Policy Uncertainty | Hedge demand | 5-Y, 5-Y inflation swaps; Economic Policy Uncertainty (EPU) index |
Commodity Curve Carry | Roll yield from contango/backwardation | (Spot – 3-M future)/spot, monthly |
Physical Demand Factor | Jewelry & tech cycles | Shanghai Gold Exchange premium, Indian import duty-adjusted price |
ETF & Speculative Flows | Hot money | GLD daily AUM delta; Managed-money net longs from CFTC |
Mining-Cost Proxy | Long-run value floor | GDX all-in sustaining cost (AISC) index changes |
Risk-Aversion Regime | Safe-haven bid | VIX percentile, MOVE index, CDS index spreads |
3. Data Challenges & Solutions
- Lag disparity. GLD flows are t-1; CFTC positions are t-3, Shanghai premiums are t-1 Asia close. Align by event timestamps and use Kalman smoothing for missing points.
- Unit harmonization. Mix of %, basis points, tonnes, dollars. Convert to z-scores over 3-year rolling windows.
- Structural breaks. Gold’s linkage to real yields strengthened post-2008 QE era. Apply state-space break tests (e.g., Bai-Perron) and refit factor loadings across detected regimes.
4. Model Construction: From Factors to Expected Returns
4.1. Multi-Factor Risk Premia Regression
Predict weekly log-returns Δln Pₜ₊₁ = β·Fₜ+ ε. Use Newey-West errors (5 lags) to tackle heteroskedasticity.
Add interaction terms:
- Real-yield × dollar strength captures policy divergence episodes (e.g., 2022 strong USD + rising real yields smashed gold).
- Inflation expectations × ETF net flows catches reflexivity in inflation-hedge narratives.
4.2. Non-Linear Augmentation
Gold’s reaction to VIX is convex: mild equity drawdowns often see gold sell off (liquidation for margin), but severe crises see steep gold rallies. Fit spline regressors or piece-wise GAM (generalized additive model) for risk-aversion factor.
4.3. Regime-Switching Layer
Implement a 2-state Markov-switching model on the residuals:
- Hedge Regime (H): Negative β_real-yield, positive β_inflation, higher σ.
- Carry-like Regime (C): Weak macro sensitivity, dominated by curve carry and physical demand factors.
Transition probabilities driven by macro volatility and Fed balance-sheet growth. This layer lets you increase gross exposure during Hedge states when Sharpe >1 historically.
5. Portfolio & Trade Design
- Instrument Choice. Trade COMEX futures (micro and standard), Loco London spot via XAUUSD, or GLD/IAU ETFs depending on mandate.
- Position Sizing. Convert model’s expected Δ% to target risk using w = (μ / σ_target) / EVT. EVT = expected-tail volatility via Cornish-Fisher VaR (captures gold’s skew).
- Execution. Gold futures show U-shaped intraday liquidity—peak around CME open (13:20 GMT) and London PM fix (15:00 GMT). Slice orders via time-weighted algo, tapering size during China lunch break.
- Leverage Constraints. Spot XAUUSD at retail brokers offers 50-100×. Institutional desks typically cap at 5×. Encode guardrails into the order manager.
6. Risk & Stress Testing
- Real-Yield Shock. Shock TIPS yields +75 bp, re-price expected gold return via beta.
- Liquidity Crunch. Simulate GLD outflows of −200 tonnes in 5 sessions; propagate to spot via NAV arbitrage bands.
- Central-Bank Dump. Assume IMF offloads 400 tonnes; feed into supply factor and observe drawdown.
- Variance Gamma Jumps. Fit a VG model to weekly returns; embed jump intensity into VaR.
7. Ongoing Factor Governance
Governance Step | Frequency | KPI |
Information Coefficient (IC) | Weekly | >0.05 target |
Turnover vs. Slippage | Monthly | Slippage/Tot α <10% |
Factor Crowding | Monthly | Herfindahl of CTA holdings in gold futures |
Model Risk Review | Semi-Annual | Backtest vs. live drift; scenario alignment |
What-if: If central-bank digital currencies reduce USD hegemony, dollar factor weakens. Your governance cadence should catch declining IC early.
8. Conclusion
A gold factor model must sit at the nexus of macro-monetary regimes, commodity market microstructure, and investor psychology. By weaving together real-yield dynamics, cross-asset volatility signals, curve carry, and physical flows, you capture the metal’s three identities—store of value, inflation hedge, and risk barometer. Continuous regime recognition, data hygiene, and disciplined risk budgeting convert that conceptual richness into tradable alpha.