Advanced Strategies in Cycle Analysis for Stock Market Success
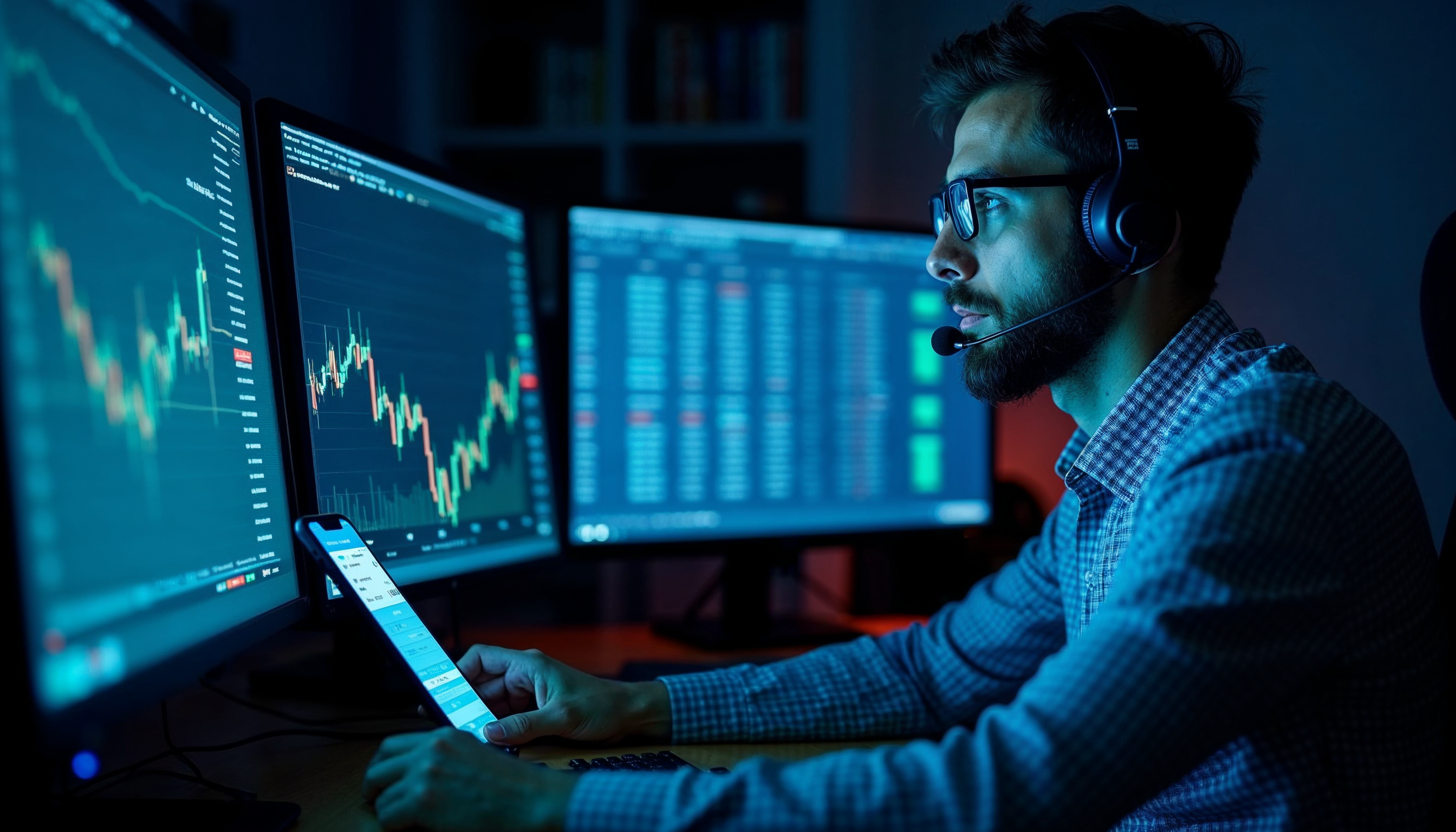
Introduction
Cycle analysis is a sophisticated approach used by traders and analysts to decipher recurring market trends. By delving deep into the cyclical nature of the stock market, investors can adopt advanced strategies that lead to sustained trading success.
Deep Dive into Market Cycles
Seasonal Cycles: Seasonal cycles, influenced by calendar events, affect market behavior predictably each year. Notable examples include the January Effect or holiday shopping periods impacting retail stocks. Investors analyze historical seasonal trends to forecast future market movements accurately.
Economic Cycles: The broader economy significantly influences market cycles through phases of expansion, peak, contraction, and trough. Economic indicators such as GDP, unemployment rates, and consumer sentiment are vital for understanding these cycles. Investors aligning their strategies with economic cycles achieve better long-term outcomes.
Sector Cycles: Different market sectors move cyclically due to industry-specific factors. Recognizing these cycles allows investors to rotate investments strategically, benefiting from sectors positioned to outperform during specific cycle phases.
Sophisticated Analytical Techniques
Wavelet Analysis: Wavelet analysis provides flexibility in detecting cycles of varying durations. Unlike traditional Fourier analysis, wavelet analysis captures non-stationary cycles, enabling investors to adapt quickly to changing market conditions.
Time-Series Decomposition: Breaking down stock market data into trend, cyclical, seasonal, and irregular components, time-series decomposition provides clarity on underlying movements. Investors gain detailed insights into market dynamics, improving forecasting accuracy.
Dynamic Mode Decomposition (DMD): DMD is a data-driven technique that models complex systems like the stock market. By extracting dominant market dynamics, DMD helps traders anticipate changes accurately and adjust strategies promptly.
Integrating Cycle Analysis with Other Strategies
Cycle Analysis and Technical Indicators: Combining cycle analysis with indicators like RSI, MACD, and Bollinger Bands enhances predictive power. For instance, aligning cyclical peaks with overbought conditions in RSI signals potential market tops.
Machine Learning Approaches: Integrating machine learning models with cycle analysis allows investors to predict stock trends more precisely. Machine learning algorithms trained on historical cycle data can dynamically adjust predictions based on real-time market inputs.
Real-world Examples of Cycle Analysis
The Business Cycle and Investing: Historically, certain sectors like consumer staples and utilities outperform during recessionary periods, while technology and industrial stocks excel in expansions. Investors applying cycle analysis strategically position their portfolios for these transitions.
Using Elliott Wave Theory: Elliott Wave theory segments market movements into predictable waves reflecting investor psychology. Traders skilled in this cycle-focused analysis can capitalize on market movements by timing trades based on identified wave patterns.
Risks and Mitigation Strategies
Cycle analysis, despite its strengths, faces challenges including unpredictable events and irregular market behavior. Investors should employ comprehensive risk management strategies, such as setting stop-loss orders and diversifying across asset classes, to mitigate risks associated with cyclical inaccuracies.
Conclusion
Advanced cycle analysis equips investors with the tools needed to anticipate market trends accurately. By mastering sophisticated analytical methods and integrating these insights with complementary strategies, traders can significantly enhance their potential for sustained market success.